Three mistakes causing your "data-driven" strategies to fail to create breakthrough performance
(And how to fix them)
After two decades working for companies of all sizes—from rapidly growing startups of six people to companies with hundreds of thousands of employees—, I’ve come to the conclusion that organizations tend to fail to become “data-driven” (or, how I prefer to say, “evidence-based”) for three primary reasons.
We’ll get to them, but first the good news: if you think your company is behind in its ability to compete in analytics, keep in mind that even organizations that dominate their fields and are known for their industrial-strength analytics from time to time suffer from the same weaknesses.1 Moreover, the competitive advantage these industry leaders developed due to the prohibitive cost of technology and difficulty consolidating structured and structured data is quickly disappearing. As a byproduct of technological advancements, now every firm in every industry has the ability to leverage data imbued with relevance and purpose to support better decision-making.
Here are the three mistakes to avoid:
1. You ask your data team to go on “fishing expeditions”
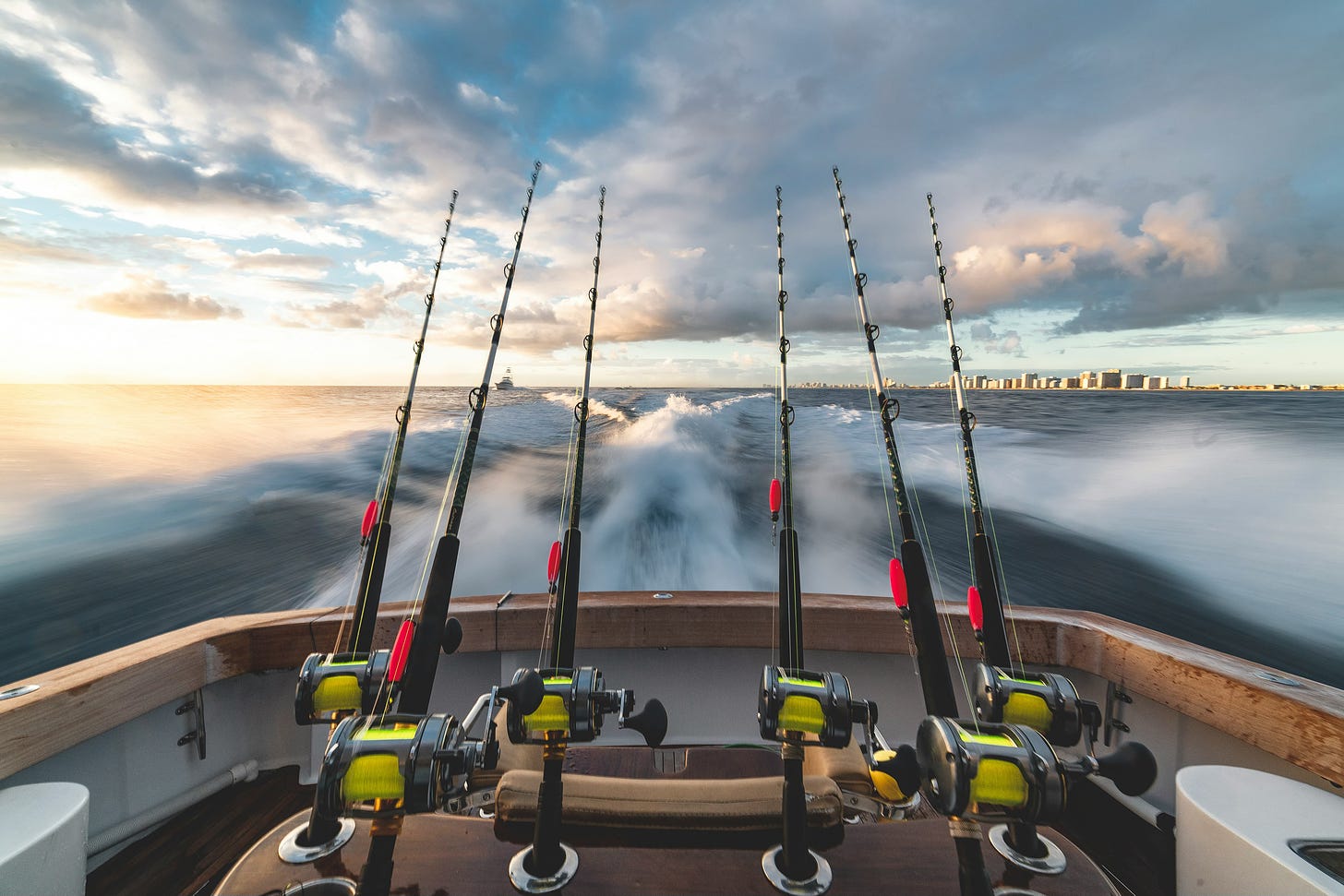
Imagine that sales are going down. If you ask your data analysts to go figure out what’s going on without additional context, chances are they’ll look at every variable they can possibly get their hands on at once. And they’ll probably find relationships that don’t really exist (the same principle of flipping a coin multiple times and incorrectly concluding that a run of consecutive heads or tails means the coin is biased).
Solution: Make sure you have at least one member of your data & analytics team that is a hybrid of statistician, analyst, communicator, and trusted advisor. Give this individual an incentive to speak up when a problem brought to the analytics team isn’t well formulated or need to be further distilled into a set of hypotheses that can be properly tested.
2. You ignore the issues caused by data silos
In one of my projects, I worked with a telecommunications company that had excellent data about subscriptions and cancellations, as well as data usage and quality of service. However, due to departmental silos, the business had no way of cross-referencing these two sources of data to allow an understanding of how usage behavior and signal strength affected customer churn.
As a result, the company was able to create wonderful dashboards with beautiful charts to display its vast collection of historical data, but failed to develop an understanding of what was causing the business to lose an alarming number of subscribers every month.
Solution: First, determine the root cause of the problem. It may be technical (data that is not naturally congruent or easy to integrate), or, as happened in many of my projects, primarily caused by politics (internal groups being overly protective of their data and finding excuses not to share it).
The technical problem is getting easier and easier to solve with cloud-based tools that facilitate integrating data across sources. The internal politics issue may require escalation to the C-suite. Be specific about the business problems that could be solved and opportunities that could be exploited if the barriers to consolidating information from various sources (e.g., online search, customer complaints, commercial transactions) are removed.
3. You only use the data when it agrees with your intuition
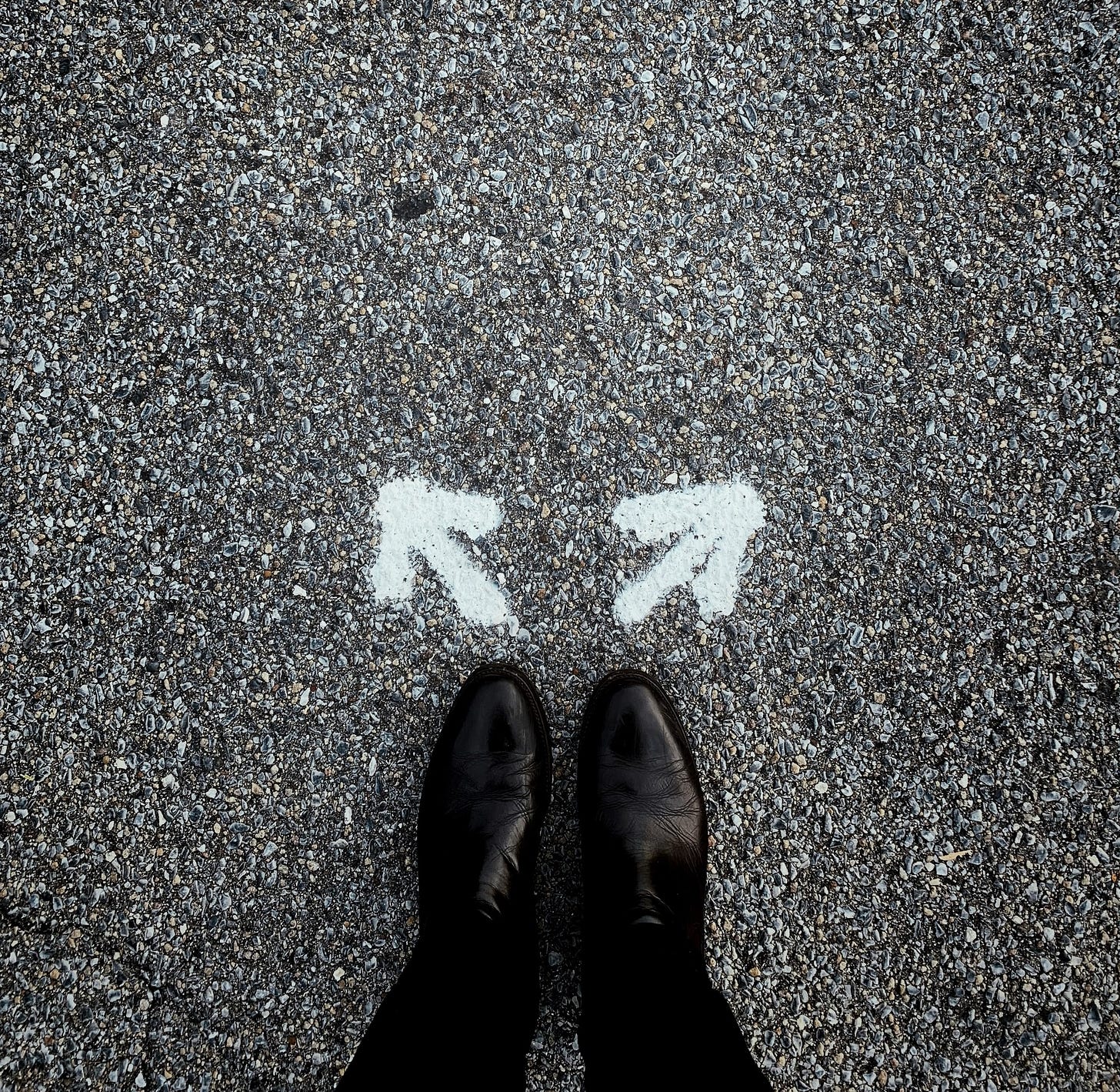
Many decision-makers only accept data that validate their own conclusions. In a project to identify fraud in a marketplace, an executive had his own intuition about what types of activity represented fraudulent behavior or not. After analyzing the data, it became clear that some of the activities that were considered legitimate also pertained to fraud. Rather than accepting the evidence, the executive kept asking for more and more analyses, constantly delaying important decisions necessary to curb the fraudulent behavior.
The solution: If you’re a senior executive, help change the decision-making culture by openly recognizing when data have disproved one of your hunches, and allowing your opinion to be overridden. lf you’re not part of the leadership team, see if you can pick a quant-friendly leader to help teach the organization the habit of asking, “What do the data say?”
In parallel, update processes and create simple, understandable tools for people on the frontlines to view analytics as central to their decision-making.2
As a data science consultant, I lost count of the times when I heard a customer say, “Oh, but we have such limited data!”—only for me to realize that they had better data that many of their competitors that are ahead in the use of sophisticated modeling to sharpen their marketing, risk management, or operations.
As I wrote in a past article, imperfect data is often good enough. Companies succeed in leveraging data to improve decision making not because they have more or better data, but because they have leadership teams that ask the right questions and maximize cross-functional cooperation to learn from the available data.
To better compete on analytics, focus on helping your leaders and employees become more evidence-based in their thinking, more open to sharing the mountains of data trapped in department silos, more knowledgeable on how to frame and test hypotheses, and more willing to conduct experiments and incorporate evidence-based insights into their decision-making.
While I can’t share proprietary information about my consulting work here, it’s not difficult for anyone to find examples of market leaders failing to translate big data into meaningful business insights. As an example, in my mailbox I currently have a message from a retail business that touts itself as a leader in analytics and has more than a decade of my purchase data. In the message, they’re advertising a product that targets parents of teenagers despite the fact that I don’t have any children.
For the best results, make sure any data & analytics project starts from a specific problem or potential opportunity to be transformed in a hypothesis that can be tested, ideally in a matter of weeks. For instance, in one of my projects, the hypothesis was, “If we separate our customers by segment and structure our sales conversations accordingly, we’ll see an increase in sales.” Using ad-hoc data extractions exported to a cheap cloud storage repository, my team quickly developed a clustering model that segmented existing customers based on their demographics and purchase history. The company then used a controlled experiment in which salespeople adopted different presentation styles to communicate with customers in each segment. After validating that the approach helped close more deals, the business equipped the entire sales team with scripts that immediately improved their sales interactions and win rates.